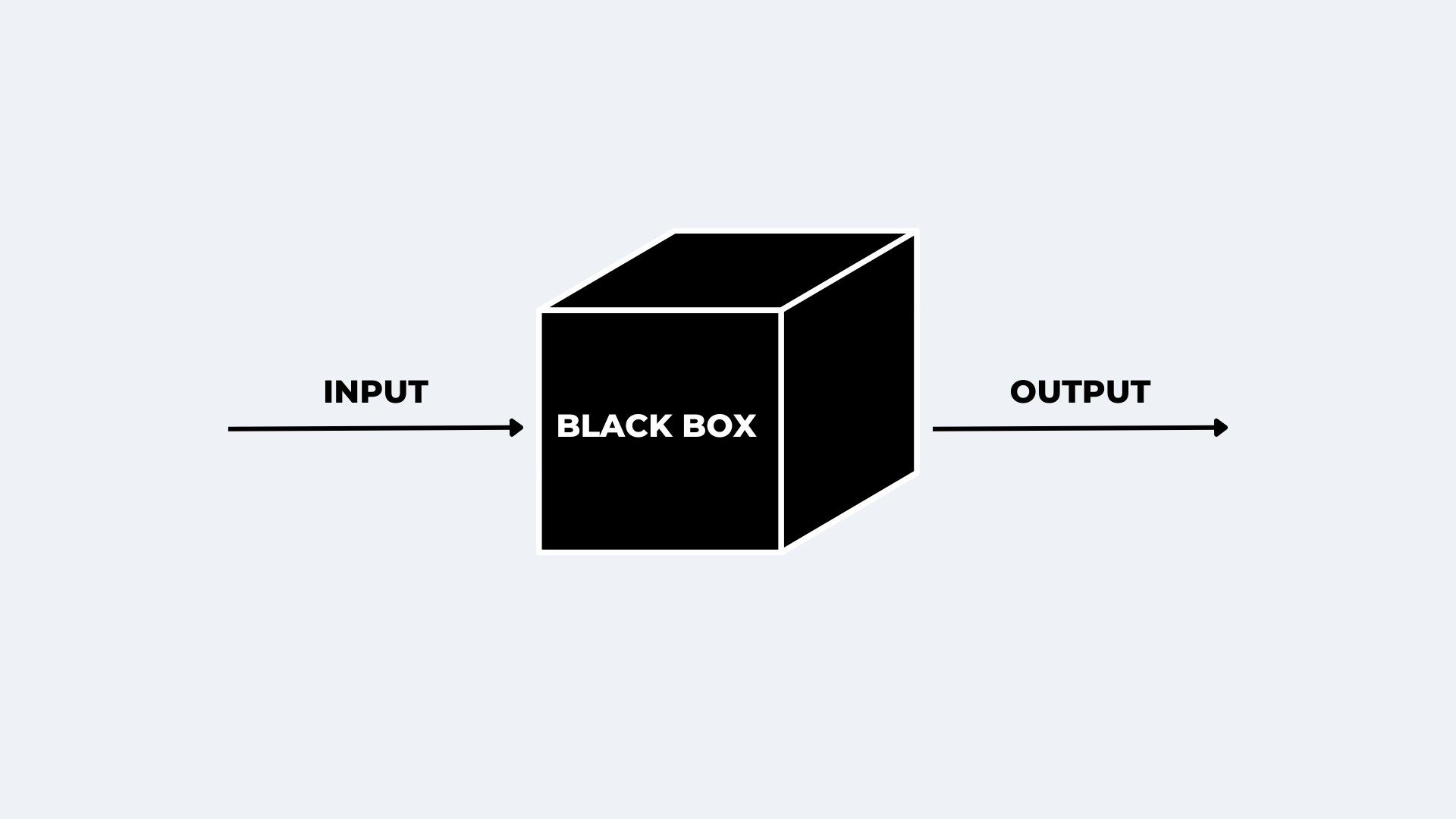
The Undared World of Artificial Intelligence
Although the big language models that form the basis of artificial intelligence today have shown an extraordinary success in understanding and producing human language, the decision -making processes of these models are largely the mystery of the decision -making processes. We can see which commands are given to a model and what kind of responses it produces; However, it is still unclear to understand how these answers are created.
This unknown, leads to trust problems in artificial intelligence practices. It is difficult to predict the tendencies of models to produce incorrect information. In addition, some users can not be fully explained in some cases why the “Jailbreak” techniques applied by some users to overcome security measures.
Anthropic researchers took a big step to analyze this complex structure. Inspired by FMRI technology used to examine the human brain, the team developed a new tool to understand the internal functioning of large language models. This technique reveals which processes of artificial intelligence models are activated by mapping their “brains”.
Researchers who applied this new tool to the Claude 3.5 Haiku model showed that artificial intelligence can make planning and logical inferences even if not conscious. For example, when a poem was given a task of writing, it was observed that the model identified the compatible words in advance and then made sentences in accordance with these words.
Another remarkable finding of the research was the logic structure of language models in multi -lingual work. Instead of using separate components in different languages, the Claude model works using a common conceptual field for all languages. In other words, the model first enters into a reasoning process through abstract concepts and then turns this idea into the desired language.
This finding opens new doors about how multilingual artificial intelligence can be made more efficient. This approach, especially for companies that develop global -scale artificial intelligence solutions, can ensure that models work more consistent and faster.
Why is the opening of the black box important?
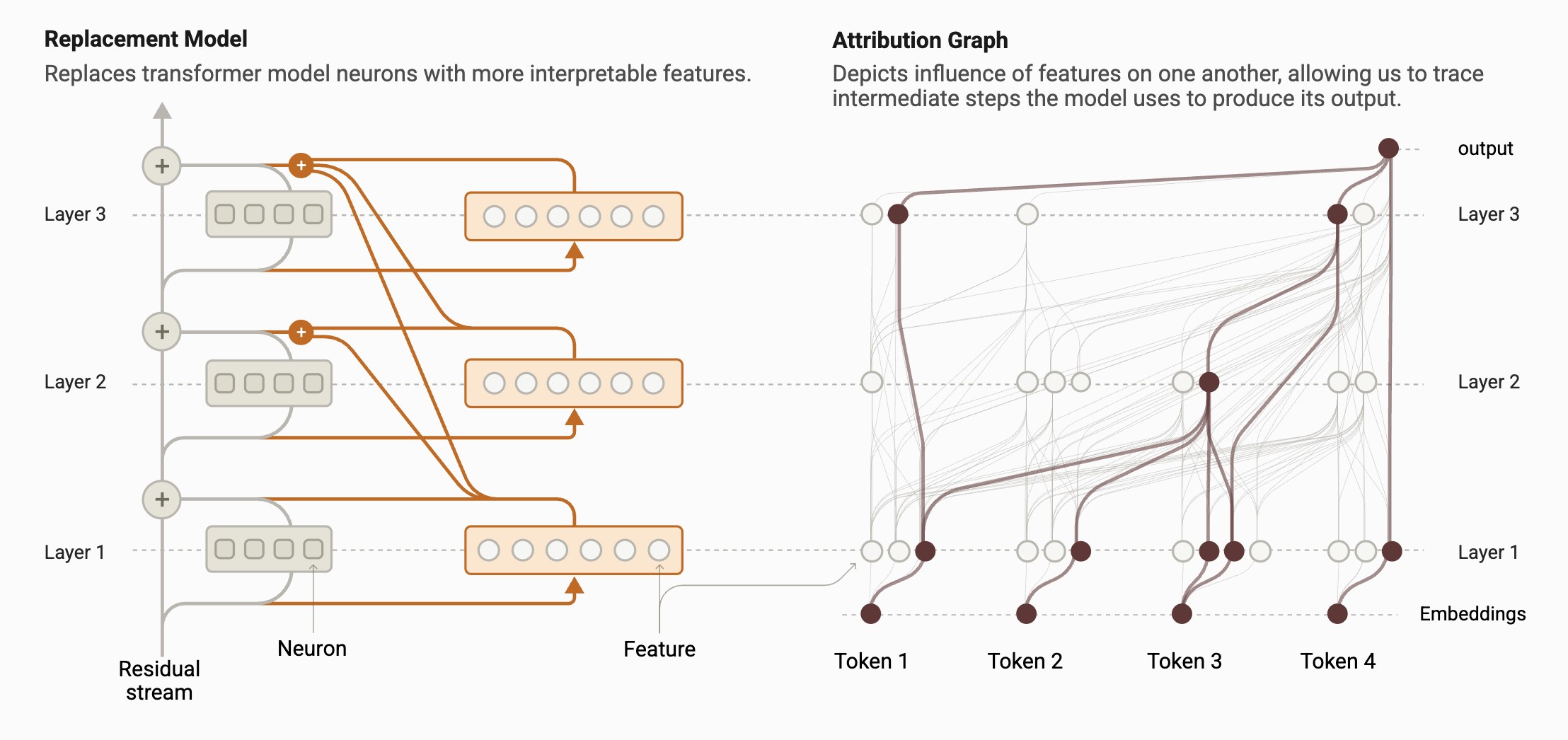
On the other hand, according to some experts, this mysterious structure of LLMs is not a very big problem.
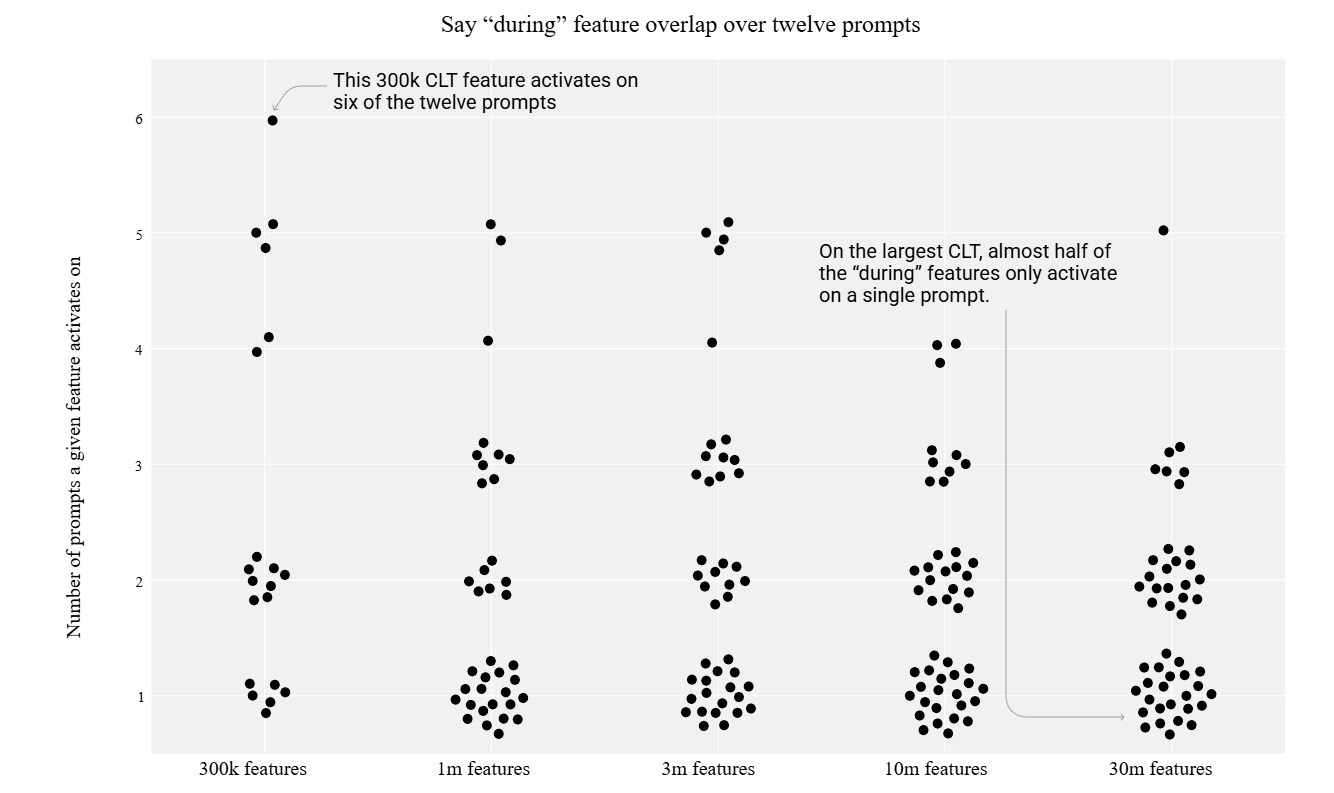
However, the problem with large language models (LLMs) is that the methods of accessing outputs are quite different from the way people do the same tasks. Therefore, they can make mistakes that a person is unlikely to do.
Cross-Layer Transcoder (CLT) Approach
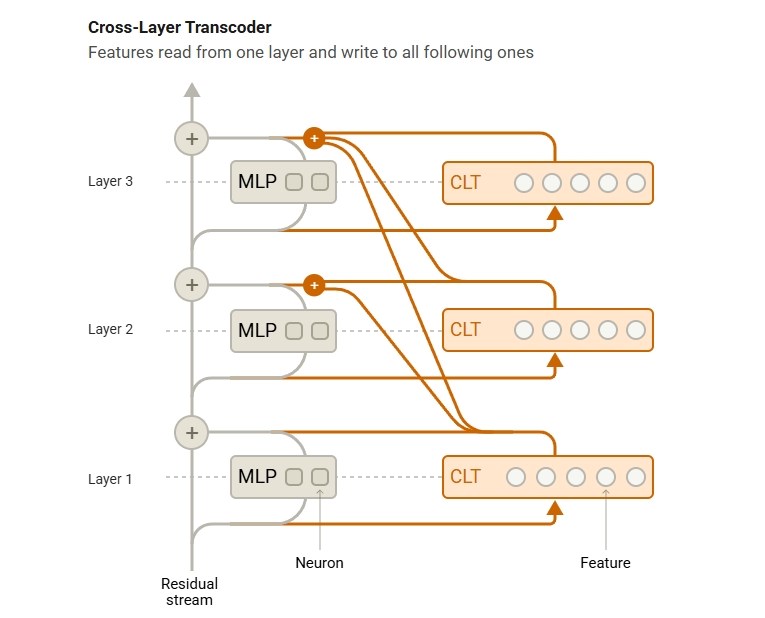
Anthropic has developed a completely new model to overcome this problem: Cross-Layer Transcoder (CLT). The CLT analyzes the artificial intelligence model at the level of interpreted features, not at the level of individual neuron levels. For example, all the shootings of a particular verb, or all terms, which mean “more”, can be considered as a single set of features. In this way, researchers can see which neuron groups work together while performing a particular task of the model. In addition, this method provides researchers the opportunity to follow the reasoning process of the model throughout the layers of neural network.
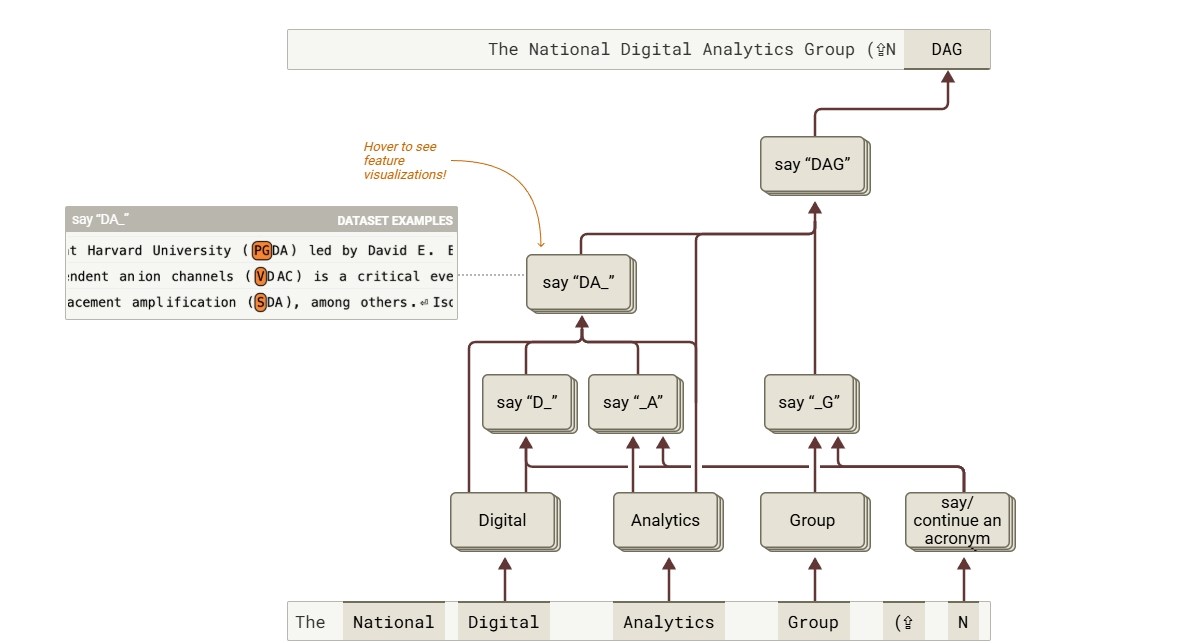
Anthropic also stressed that analyzing the circuits of the network is quite time -consuming. Even for short inputs consisting of “dozens of words”, he can take a few hours of a human expert. How the method can be scaled to much longer inputs remains unclear. However, CLT is an extremely important step to open Pandora’s box.